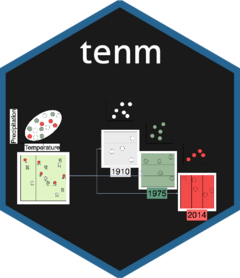
Function to compute the covariance matrix of an ellipsoid niche model.
Source:R/cov_center.R
cov_center.Rd
Computes the covariance matrix, niche centroid, volume, and other ellipsoid parameter based on the values of niche variables from occurrence points.
Arguments
- data
A data.frame or matrix containing numeric values of variables used to model the niche.
- mve
Logical. If
TRUE
, computes a minimum volume ellipsoid using thecov.mve
function from the MASS package. IfFALSE
, uses the covariance matrix of the input data.- level
Proportion of data to be used for computing the ellipsoid, applicable when mve is
TRUE
.- vars
Vector specifying column indexes or names of variables in the input data used to fit the ellipsoid model.
Value
A list containing the following components:
centroid
: Centroid (mean vector) of the ellipsoid.covariance_matrix
: Covariance matrix based on the input data.volume
: Volume of the ellipsoid.semi_axes_lengths
: Lengths of semi-axes of the ellipsoid.axis_coordinates
: Coordinates of ellipsoid axes.
Examples
# \donttest{
library(tenm)
data("abronia")
tempora_layers_dir <- system.file("extdata/bio",package = "tenm")
abt <- tenm::sp_temporal_data(occs = abronia,
longitude = "decimalLongitude",
latitude = "decimalLatitude",
sp_date_var = "year",
occ_date_format="y",
layers_date_format= "y",
layers_by_date_dir = tempora_layers_dir,
layers_ext="*.tif$")
abtc <- tenm::clean_dup_by_date(abt,threshold = 10/60)
future::plan("multisession",workers=2)
abex <- tenm::ex_by_date(abtc,train_prop=0.7)
future::plan("sequential")
mod <- tenm::cov_center(data = abex$env_data,
mve = TRUE,
level = 0.975,
vars = c("bio_05","bio_06","bio_12"))
# Print model parameters
print(mod)
#> $centroid
#> bio_05 bio_06 bio_12
#> 217.10256 54.69231 1106.07692
#>
#> $covariance
#> bio_05 bio_06 bio_12
#> bio_05 731.3050 456.5061 -249.8502
#> bio_06 456.5061 418.6923 987.1559
#> bio_12 -249.8502 987.1559 152235.5992
#>
#> $niche_volume
#> [1] 14127753
#>
#> $SemiAxis_length
#> a b c
#> 28.44591 99.38665 1192.98935
#>
#> $axis_coordinates
#> $axis_coordinates[[1]]
#> bio_05 bio_06 bio_12
#> vec_1 233.5857 31.50945 1106.254
#> vec_2 200.6194 77.87517 1105.899
#>
#> $axis_coordinates[[2]]
#> bio_05 bio_06 bio_12
#> vec_1 298.1029 112.282107 1105.835
#> vec_2 136.1022 -2.897492 1106.319
#>
#> $axis_coordinates[[3]]
#> bio_05 bio_06 bio_12
#> vec_1 215.1587 62.44309 2299.03951
#> vec_2 219.0465 46.94153 -86.88566
#>
#>
# }